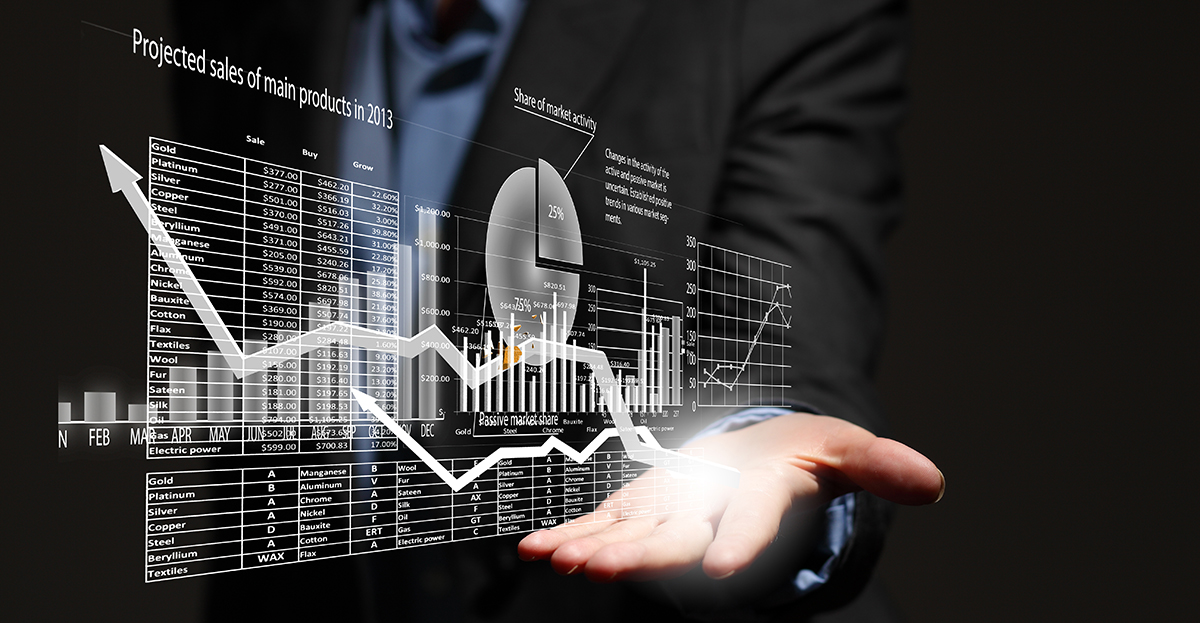
Why Using More People Analytics Could Have Unintended Consequences
Use of data and analytics impacts almost every area of business today. And the trend is for this to continue to increase, as adoption in many areas is in the early stages of maturity. Most of the discussion of analytics is focused on the positives—that is, the huge potential for using data and analytics to make better decisions, improve efficiency, make better products, etc.
Like most things in life though, there are the possibilities of downsides, of unintended consequences or side effects of using more data and analytics in business. While working on i4cp's latest research study on people analytics, I noted a couple of examples of this playing out elsewhere, which led me to consider unintended consequences with people analytics as well.
Example: China’s Big Plans
While most of us are comfortable to varying degrees with the government and private organizations having bits of data about us, the government of China is moving to take this to a new level—a digital reboot of their earlier attempts at social control (see Wired magazine’s January 2018 story titled "Inside China's Vast New Experiment in Social Ranking" and the Wall Street Journal's November 28 article "China’s New Tool for Social Control: A Credit Rating for Everything").
China is developing a broad scoring system for citizens that goes well beyond the FICO credit scoring system those in the U.S. are used to when buying a house or car. In short, the Chinese government's State Council is establishing a "social credit" system and that will serve as a nationwide tracking system to rate the reputations of everyone—individuals, businesses, and even government officials. The goal is for every Chinese citizen to be trailed by a file compiling data from public and private sources by 2020.
The Wired article notes, "For the Chinese Communist Party, social credit is an attempt at a softer, more invisible authoritarianism." That may be true, and the system will no doubt have some benefits in enabling more commerce, increasing trust between some entities, and more. The system will gobble up data from sources that range from bill payment, adherence to traffic rules, volunteer activities, shopping habits and more, and in turn determine eligibility for much of what people want and need to do in life: access to social services, loan rates and amounts, school admission and scholarships, eligibility for a range of jobs, options to travel abroad, and much more.
While some of the data sources and intended uses of such a social credit system might disturb U.S. sensibilities (e.g., being penalized for "spreading online rumors" or violating China's family planning limits), it doesn't take long to think of even worse unintended, negative consequences of such a broad-ranging system. After all, it is one thing to blacklist someone from flying who might be a suspected terrorist or other safety threat. But what if an individual businesswoman has a low social credit score because she fell behind on paying a utility bill, doesn't visit her elderly parents often enough, or has friends with low scores? That last input to the system raises several questions in particular, as people will change their "friending" behavior in order to raise or not lower their score, and even try to make artificial connections to game the system's algorithms to help their score (this is already happening).
Example: Major League Baseball
While this system in China is developing and it is unclear how Orwellian the results will be, such an extreme example is not the only one I found in which well-intentioned use of data and analytics can and does have unintended, negative consequences. Major League Baseball has for decades been a primary case of the use of big data and analytics improving performance and results. So-called sabermetrics (named after the Society for American Baseball Research) have gone from being a niche interest of geeky baseball statheads, to being popularized by authors such as Bill James, and to some extent normalized by the book and movie Moneyball. Aside from old-school scouts who prefer their gut-instincts, few in baseball would dispute the benefits of an ever-increasing sophistication in the use of data and analytics to improve all aspects of the game and ultimately the business results for the teams that focus on it.
That said, an analysis of recent trends brought to light some unintended, negative consequences from the use of so much data and analytics in baseball. The 2017 baseball season saw several overall major league records broken. Many have noted that the new peak in homeruns hit per game, as well as the often related statistic of the most strikeouts per game. A less frequently cited record from 2017 was a new low in the number of complete games thrown by starting pitchers. A careful look at the trend data shows that this has dropped dramatically in the past two decades, the same time period when the use of data and analytics has surged across the baseball world. With fewer complete games being thrown by starting pitchers, teams must rely ever more on relief pitchers. This was already trending since the 1970s, but the recent reliance on data and analytics has hastened the development of all manner of relief specialists. The result in 2017 was a record average of 4.22 pitchers used per team, per game. That number has increased in each of the past few years.
The problem is that when more relief pitchers are used, the length of the games significantly increases. This is because when a relief pitcher comes in they have to run in from the bullpen, pitch a few final warm-up pitches, etc. Sure enough, 2017 saw a record for this as well: the average game clocked in at three hours, eight minutes, which was barely longer than the previous high of three hours, seven minutes in 2014. But from 1987 to 2012 the average game length was a bit under three hours, and from the mid-1950s through the 1970s the average was only about two and half hours.
Unfortunately, this result runs counter to the desired goal of decreasing the length of Major League games. Understanding that fans have grown weary of games that run longer and longer, the Commissioner's office has been recently instituting subtle changes to speed up gameplay (e.g., limits on the time pitchers and batters can take between pitches, automating intentional walks, and more). But the ever-increasing use of data and analytics, with its impact on pitching and other aspects of the game, is causing the trend to go in the other direction.
Avoiding Similar Issues with People Analytics in Business
Examples like these from China and baseball should give organization leaders pause amidst their excitement about the prospects of using data and analytics to make better decisions, improve efficiency, and more. People analytics leaders in HR are not immune to this consideration: What could the unintended, negative consequences be of gathering ever more data about your organization's people, analyzing that data, and using the results as input for decisions and strategy, or in the case of more mature organizations, as a driver of HR strategies?
As part of our recent i4cp research on people analytics, we interviewed nearly a dozen people analytics leaders at large organizations, and asked each of them that question. There are of course concerns with data security and privacy, and some noted that an over-reliance on data and analytics could result in a backlash amongst employees who believe they are being reduced to numbers (perhaps not on the level of China's plans, but the concern remains).
One concern voiced was making sure your people analytics function doesn't fall into the trap of wasting time on irrelevant metrics just because you can measure something. Organizations can guard against this by making sure that for each people analytics initiative or project, there is a clear business question you are trying to answer or business outcome you are trying impact. Ask yourself: What change in behavior will we recommend after getting the data back and analyzed? How will the results of an investigation move one or more needles and impact business results?
Data in performance management in organizations can have unintended consequences: a negative impact on employee morale, inequality between departments, or perverse incentives to focus exclusively on one's own KPIs to the detriment of collaborating with peers. Savvy organizations are alleviating this issue by following the next practice identified in i4cp's recent study on collaboration, which found that high-performance organizations are 5.5x more likely to have performance goals for teams that reinforce the importance of collaboration.
A heavy reliance on data and algorithms in talent acquisition can help alleviate the pain of an ever-increasing flood of applications. But an over-reliance on, or poor design of, such algorithms, coupled with limited human interaction in the process, can have several unintended consequences including impact on the organization’s employer brand and missing well-qualified candidates who don't fit the pre-programmed models.
But perhaps the most interesting example came from a couple of organizations that clearly have mature people analytics functions. Such teams are pursuing predictive analytics that help organizations to understand what is likely to happen given past similar situations. While such projects often provide managers and business leaders with invaluable insights to help them make decisions faster and better, the data can at times have unintended consequences.
For instance, data analysis might result in a predictive turnover model, something that indicates that employees with certain characteristics are more likely to leave the organization than others. If these are otherwise valuable employees, such insights can be a warning sign to a manager to pay particular attention to the employees' immediate needs, desire for growth in the organization, and so on. While there can be false positives, the idea is to help managers get ahead of the potential loss of a valued team member.
But such data could also have the unintended consequence of leading a manager to do the opposite. If the data says some individuals are more likely to leave, the perception might be that they are lost causes and not worth special attention. A manager might not assign them to important or interesting projects fearing they will leave soon, creating disruption. Or perhaps fewer resources will be dedicated to such employees' training and development.
As one leader noted, results from predictive analytics should be taken as insights, but not as absolutes. They are an indication of what is more likely to happen based on particular data, but due to the complexity of human behavior they are often better viewed as estimates rather than strong predictors of future outcomes. Coaching managers and other consumers of such predictive data to consider what it does and does not mean is critical to them using it as input for sound decisions rather than costly mistakes.
Data and analysis can shape behavior for good and ill. To avoid unintended negative consequences in the use of people analytics, HR leaders must be careful and intentional every step of the way. Fortunately, as our latest research indicates (available soon to i4cp members), with significant advances happening in the maturity of people analytics functions, organizations are increasingly well-poised to reap the benefits while avoiding any downsides.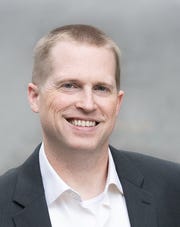