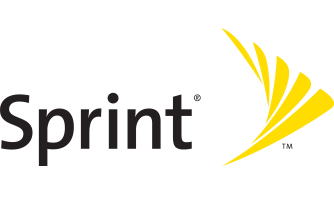
Case Study: How Sprint Uses Predictive Analytics
Sprint, an i4cp member organization, is one of four major players in the fiercely competitive U.S. wireless communications market. Innovation, even within the human resources department, is at a premium there, and Sprint’s ability to accurately measure and tie programs to business outcomes has allowed for the creation and maintenance of innovative programs that have helped fuel the highest rating in customer loyalty among U.S. national carriers, and has made it the only national carrier to see consistent improvement in this area since 2010 (The American Customer Satisfaction Index, 2012).
i4cp has released a case study – the first of a four-part evidence-based HR series sponsored by i4cp's Evidence-Based HR Exchange – that examines Sprint’s efforts in moving from observational to predictive analytics, as well as discussing the most important lessons from their employee data analysis journey.
Evidence-based HR is an approach to strategically managing and measuring talent to help human resources more effectively drive the business. It enables leaders to know which human capital practices are most effective and what needs to be reexamined, and provides the platform necessary to prove the real business impact of talent initiatives. But an evidence-based approach to HR is easier said than done.
Since beginning the task of building this level of analytics capability in February 2011, Tom Sullivan, Manager for Workforce Analytics at Sprint, has focused primarily on the bottom line of productivity with the question, "How do we know that the workforce is more productive today than they were yesterday, a month ago, a year ago?”
To help answer Sullivan’s questions, Sprint launched an enterprise-wide survey of their workforce, which they then combined with performance indicators available from certain departments (most notably customer management and retail sales) to create entirely new ways of interpreting the data.
Sullivan and Scott Jensen, Workforce Optimization Manager, used these analytics to get a clearer picture of what factors might enhance employee productivity. Although Sullivan’s group was focused on the front-line, transactional employees, Jensen was able to use that work to get an indirect view of the roles that support productivity among those employees, which provided him with a better understanding of the employee lifetime value curve.
What Sullivan found in the data was that constraints on productivity were not purely driven by the employee – that is, there was a definite limit on the employee’s ability to reach a certain productivity level. “Until we can rally around the big ideas of how to remove that strain on performance,” Sullivan elaborated, “what the employee survey tells us about driving employee engagement, commitment or sentiment – and what we’re doing from a coaching perspective – [will] get limited returns. Not because your people-related programs are flawed, but because you have this downward constraint from the environment not only within the customer management space, but also in our frontline retail.”
A focus on recognizing and overcoming environmental constraints is what makes Sprint so unique in their approach to evidence-based HR analytics. Organizations will often focus on ways to improve worker productivity by finding ways to make them work faster, smarter, harder, etc. – forgetting that there is a limit to this side of the equation due systems and structure that will cap an individual’s productivity. Sprint has accounted for these operational barriers, allowing them to experiment with lifting some constraints and balance them against potential productivity gains.
The complete case study is available exclusively to i4cp members. Visit the i4cp website to learn more about becoming an i4cp member and the work of the Evidence-Based HR Exchange.